How data scientists can bolster the future of fintech industry
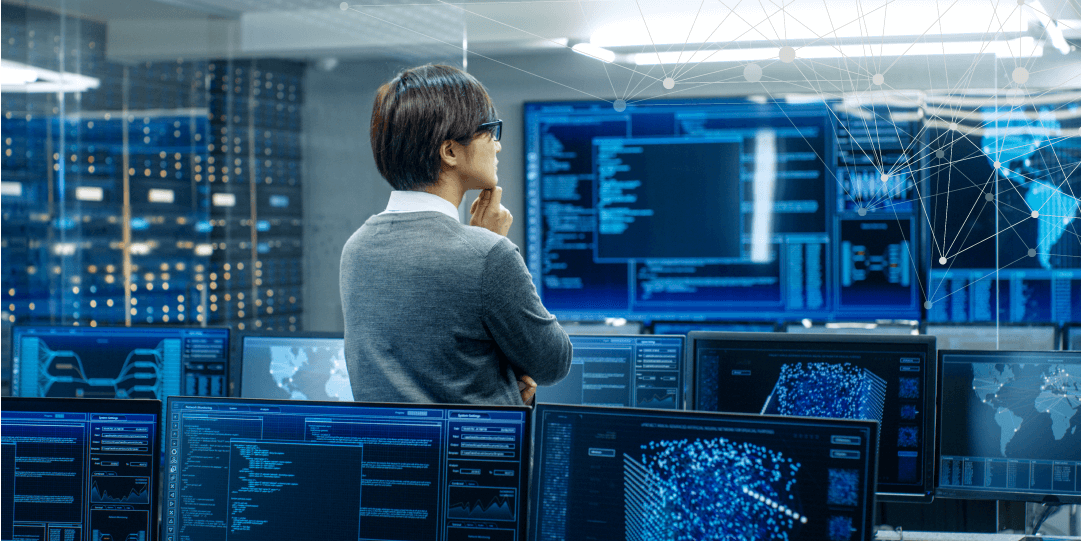
Just like the famous Gold Rush of 1849, nowadays businesses are dipping their toe in the data mine, in order to seek some value out of it. This huge chunk of data is forcing the fintech and the banking industry to unleash the power of the hidden gems that data analytics can deliver.
One can’t deny the fact that banks and financial institutions generate astronomical amounts of data in the form of customer transactional and non-transactional data. Reports state that 2.5 quintillion bytes of data are being generated every day. Have we ever thought whether this data is a promise or a peril? It is no surprise that conventional data-processing fails in managing this large volume of data and provides insights that are far from reality.
Realising the value of big data requires an analytical eye and technologies such as big data analytics, AI, and machine learning. These help in churning down data into meaningful information, thus minimising the risk decisions based on intuition.
That’s where the role of data scientist comes into the picture. A data scientist has mastered this treasure hunt as it requires them to know exactly what information to look for that will act as a booster in cross-selling and customer satisfaction. In the banking industry, a data scientist can help develop customer profiles, predict behaviours and track trends, to name a few.
According to a survey, the banking and financial services sector is the biggest market for analytics and data science professionals with 44 per cent of all jobs created in this domain. This percentage will grow in the coming years as this sector is actively using data to derive business insights and improve scalability.
The emerging role of data scientists
Over the past few years, the banking industry has achieved new heights through innovative means for evolving customer expectations of personalisation and convenience.
Earlier banks and other financial institutions used to follow a one-size-fits-all strategy where every customer was treated with the same approach irrespective of their needs and interest.
Gone are those days when customers would visit banks for every single service like depositing, checking account balance, etc. Customers now use their mobile phones to check their account balances, deposit checks, pay bills, and transfer money.
According to a research commissioned by Relay42, the data management platform (DMP), “Digital banking is growing in popularity with 53 per cent of consumers using or willing to move to an online or mobile only bank — 27 per cent have moved already, while 26 per cent are considering the switch”.
There was a time when it would take a few years to build a framework that helps banks in gathering an overall picture of their customers. Since online banking is gaining popularity, adopting big data analytics becomes all the more important. Thus, all this has given room to the new and ever-growing career of the data scientist. A data scientist helps provide meaning to the raw data and uses it to draw insights for better analysis. They help banks in establishing a 360-degree approach for their customers by the analysis of:
- Customer spending patterns
- Customer segmentation
- Implement risk management processes
- Customised product offerings
- Customer loyalty
In addition to this, data scientists help banks in designing, building and maintaining the complex data flows, tools and solutions that are needed from the bank’s data systems to analytics environments.
Moreover, data scientists tend to toil away in advanced analysis tools such as R, SPSS, Hadoop, and advanced statistical modeling. Thus, easing the process of generating valuable information from the piles of data and provide inside based on key metrics with suggestive best practices.
It can be rightly said that the fintech domain has benefited from the emergence of analytics.
The path forward
It’s high time that banks adopt big data analytics to remain relevant and profitable in this hyper-competitive business environment. Experts like data scientists will be an edge to these growing trends and will bolster the future of the fintech industry.
This career has never-ending benefits and it poses a promising future for the data science space as data has become the new oil to drive decision-making. One of the biggest challenges faced by the modern banking industry is legacy systems that aren’t equipped to handle the big data revolution. So, banks will need to align their people, processes, and technology platforms to provide highly personalised customer experience by extracting insights from data.
This article has been published in Express Computer
Read now: How data scientists can bolster the future of fintech industry
View