Democratizing Data for System Health
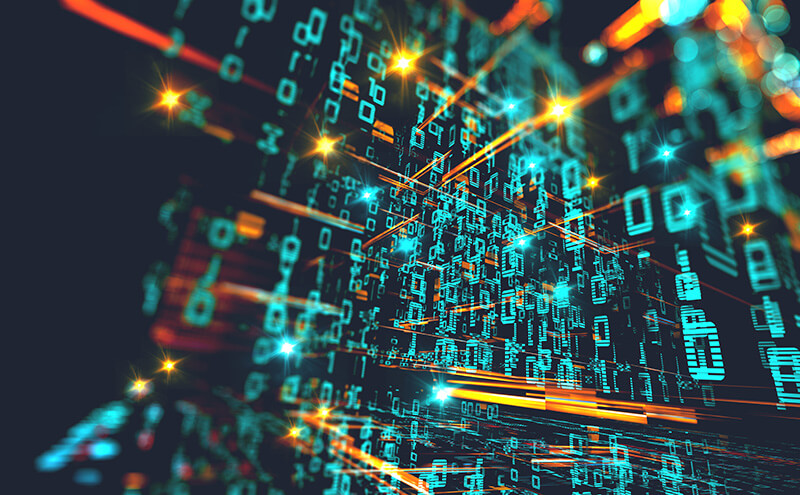
DataLab@Maveric is a hands-on scenario based learning initiative for honing real-world domain and technology skills via sandbox experiments that often result in pragmatic innovations
By the end of 2020, it is estimated that 1.7 Mb of data will be created every second for every person on the planet. That is a staggering 2.5 Quintillion data bytes each day. Take a moment to wrap your minds around that number: A quintillion is a thousand raised to the power of six (18 zeros). In fact, if those many pennies were laid out flat, it would cover the earth 5 times.
In today’s ‘data-is-the-new-oil’ economy, the pressures on data and technology teams globally arise from multiple criticalities:
- Real time event response (business events demand immediate attention),
- Data distribution (many users need to address many use cases across many systems),
- Asynchronous communication (capture data as it is created but allow applications to consume on their own pace),
- Parallel consumption (multiple parties need copies of the same data for different uses) and,
- Service Modularity (micro services architecture ensures one service does not depend on the other)
Information pattern
At Maveric DataLab, as part of one of the experiments with Apache Kafka, the team conducted deep dives on how data can be shared, re-used, secured but at the same time not be restricted. What followed was a startling insight and an opportunity. Eventually after multiple trials and feedbacks, the Maveric DataTech team came up with a unique way to truly democratize data.
Democratizing system health information includes producing a continual assessment of applications, ensuring an uninterrupted supply of services to the users, along with managing applications’ performances at optimal service levels.
Now in any secure Enterprise application, access-control is handled by IT and raw information is difficult to get, except for power users who receive data streams and are able to comprehend it. The information thereafter is used to create customized dashboards to provide different views for different user sets.
For the experiment to yield efficient results, an open, scalable, and extensible architecture is needed to address challenges in the information patterns. The Maveric DataTech team’s proof of concept uses Kafka and Apache stack of Big Data to capture and process higher volumes of data quickly and in real-time from disparate systems.
By calibrating and deploying the solution, the team reported an increased value realization across business events.
Maveric DataLabs Solution Description
The team developed an information sharing platform called ASAP (Active System Analyzer and Predictor). It collates various system and application health data from multiple application components. The collated metrics is then made available through the pub-sub model on Kafka. The content-based filtering ensures that contextual analysis of the data.
The Components of ASAP are –
- Corpus, which collates the system health information using several protocols from different environments
- Provenance, which is built on Kafka cluster which maintains messages classified into topics of contextual importance
- Ambit, which consumes the message from provenance and creates dashboard to visualize the system metrics
- Sphere, a system for contextual analysis from the derived metrics tuned to address various scenarios of monitoring and alerts
For detailed information, please get in touch with us at data@maveric-systems.com
View