2022’s Go-To Guide to Data Analytics in Banking & Financial Services
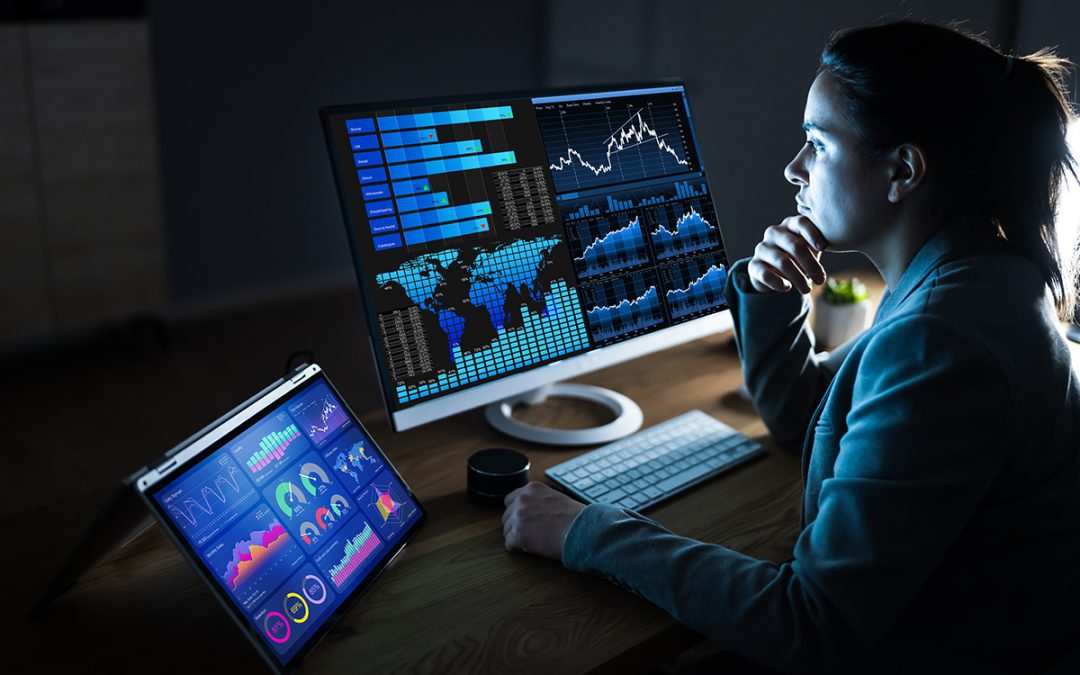
Compounding high costs of bad data are the opportunity losses banks risk with slow efforts to scale their Data Analytics function.
Not as a set of discrete projects, data analytics must evolve into an actual business discipline. The imperatives to do so are the twin drivers: advancing technologies (the exponential growth in meaningful data and available computing power) and enormous economic pressure banks face today.
Three ways data analytics generate an increase in bank’s profits.
- Amplify P&L levers (accelerate growth, enhance productivity, and improve risk control)
- Find new sources of growth (creating new business models, e.g., offering data analytics with others in the partner ecosystem)
- Deliver on the promise of a digital bank (enhanced omnichannel experience at lower costs)
Before analytics is applied to structured or unstructured sources, financial organizations have to resolve industry-specific challenges (regulatory requirements, data security, data quality, and data siloes).
Today leading banks leverage the power of analytics in more ways than one. One uses machine-learning algorithms that predict currently active customers who might drop business. Another use is to analyze competitor campaigns that curb any unnecessary discounts banks may be offering. Yet, another uses analytics to parse big data to discover microsegments in its customer base to create that next-product-to-buy.
2022 priorities for getting more out of data analytics investments.
- Post-crisis, as banking analytics use-cases increase across sales & marketing, HR, Risk & Compliance, and IT, banks will get more bang-for-their buck as they align analytics priorities to strategic vision.
- The second boost would come when managers scale analytics pilots by augmenting technical production and engineering capabilities. To succeed would mean to absorb data-driven iterations into work rhythms, something that change management programs can help in.
- The third priority concerns staffing. Individuals chosen for analytics roles (data engineers, scientists, ML engineers, e.g.) must bring a collaborative mindset.
- Financial organizations will
create value beyond the logical use-cases (digital marketing, transactional analysis, cybersecurity) by exploiting rich data sets by synching data across organizations and finding innovation breakthrough areas.
All said and done, much of these priorities would be possible when banks use robotics to eliminate 20% – 40% transactional accounting work. Not only will this allow finance teams more time for decision-making, but it also helps them gauge how best predictive analytics meshes with the performances they seek.
Banking analytics as it plays across the selling process.
Senior managers tasked with banking operations, and profitability must step back from the customer life cycle to tease out interlocks where analytics brings information and value. It is discussed below with the corresponding analytics benefit.
- Customer Identification and acquisition (acquisition analytics and campaign design)
- Customer relationship management (managing portfolio and meeting transactional needs)
- Customer cross-sell (need analysis, demography, credit history analysis, next-best-product)
- Customer retention (churn prediction, lifetime value modeling)
- Customer value enhancement and increasing wallet share (behavioral segmentation, product affinity modeling, and differentiated pricing)
As the saying goes, “Future is already here; it’s just not evenly distributed.” Banks will need to smooth out customer road maps so that each one receives high-quality and personalized relationships.
How does all this tie-up to 2022 predictions for banking data analytics?
A recent study of 10,000 companies reported that 71% are in the midst of or stand at the edge of disruption. On a 0 to 1, banking moved from 0.43 in 2011 to 0.52 in 2019. In 2020, 1 in 5 banking and payments sectors players were less than 15 years old.
The reasons are familiar – disruptive Gen Y expectations, Fintech entries, accelerated digital banking, and shifting regulation.
Given the mandate – ‘transform or make way’ – no two banks would (or should) approach data analytics the same way.
Practical steps for chasing analytics transformation and the next frontier.
The reasonable steps will remain the same as before: identify business problems, centralize data, automate processes, focus on decision making, optimize finance cycles, fight for talent, and drive continuous improvement. The innovators will turn their data analytics engines in pursuit of three strategic objectives:
- Reinforcing the core (augment existing core bank offering)
- Creating a new distribution channel (becoming a preferred partner to third parties)
- Launching innovative ventures (develop new businesses and business models)
Conclusion.
In the final analysis, as the fog lifts over the current crisis, data analytics in banking will pay dividends for players that use it for intelligent pricing, selling, retention, and intelligent prospecting.
View